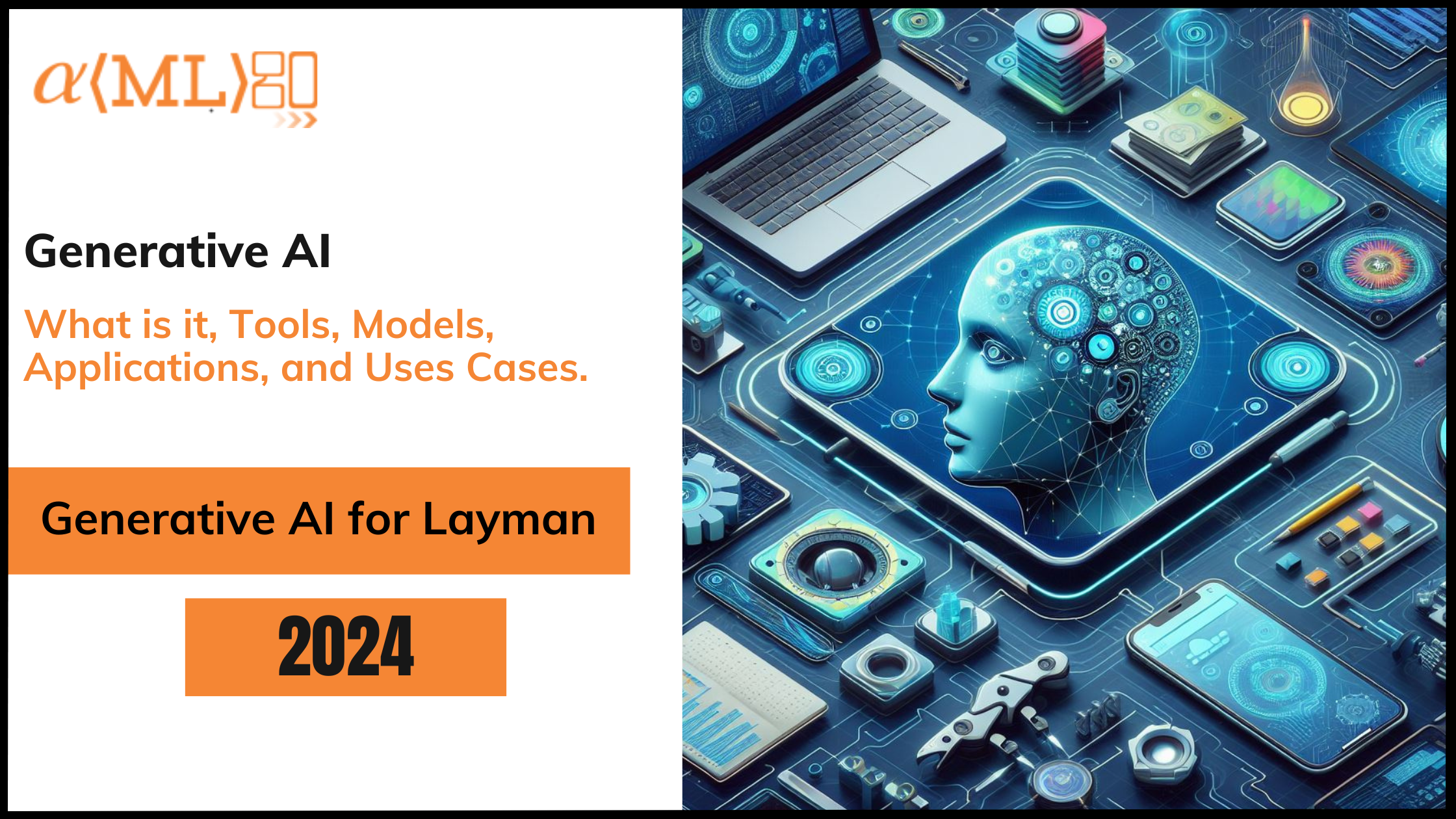
Introduction:
In the ever-evolving landscape of artificial intelligence, one of the most fascinating and transformative branches is Generative AI. This innovative technology has revolutionized the way we approach creativity and problem-solving. In this blog, we will explore the fundamentals of Generative AI, delve into the tools and models that drive its capabilities, and explore its wide-ranging applications and use cases.
What is Generative AI for a Layman:
To understand Generative AI in layman’s terms, it is a division or a class of artificial intelligence models that can generate new content, whether it be text, images, or other types of data. Unlike traditional AI models that rely on pre-existing data, generative models can create original content by learning patterns and structures from the input data during the training phase. For example – it can write emails for you and can generate new images or even videos etc.
Key Components and Tools:
- Recurrent Neural Networks (RNNs) and Transformers: These are commonly used in language-related generative tasks such as text generation and language translation.
- Convolutional Neural Networks (CNNs): CNNs are frequently employed for generative tasks involving images, such as image synthesis or style transfer.
- Neural Networks: Generative AI heavily relies on neural networks, specifically Generative Adversarial Networks (GANs) and Variational Autoencoders (VAEs). GANs consist of a generator and a discriminator, engaged in a constant feedback loop to enhance the generative capabilities of the system.
- TensorFlow and PyTorch: These are two popular deep-learning frameworks used to implement and train generative models. TensorFlow and PyTorch provide the necessary tools and APIs to build, train, and deploy complex neural network architectures.
- OpenAI’s GPT (Generative Pre-trained Transformer): GPT models, such as GPT-3,4 have gained significant attention for their language generation capabilities. These models are pre-trained on massive datasets and can perform various natural language processing tasks, making them versatile for a wide range of applications.
Generative AI Models:
- Creation of New Data: Generative AI models are designed to create new data instances that are similar to the training data they were exposed to.
- Variety of Applications: These models find applications in various fields such as image generation, text completion, music composition, and more. They can be used to generate content creatively and diversely.
- Generative Adversarial Networks (GANs): GANs are known for their ability to generate realistic images. The generator creates data, and the discriminator evaluates its authenticity, leading to a continual refinement of the generated content.
- Variational Autoencoders (VAEs): VAEs are proficient at generating diverse outputs. They work by encoding input data into a latent space, and the decoder then reconstructs this data, allowing for the generation of novel and varied content.
Applications of Generative AI:
- Content Creation: Generative AI is widely used in creative fields such as art, music, and literature, where it can autonomously produce paintings, compose music, and even generate coherent text.
- Image Synthesis and Editing: GANs are adept at creating realistic images and can be used for tasks like image-to-image translation, style transfer, and facial expression manipulation.
- Natural Language Processing (NLP): GPT models excel in NLP tasks, including language translation, summarization, and question-answering. They can understand the context and generate human-like responses.
- Style Transfer: Generative models can be used to transfer the style of one image onto another, creating visually appealing and artistic results. This has applications in photography and graphic design.
Use Cases:
- Healthcare: Generative AI is employed in medical image synthesis, aiding in generating realistic medical images for training and research purposes.
- Virtual Reality (VR) and Gaming: GANs are utilized in generating lifelike environments and characters in VR and gaming, enhancing the immersive experience for users.
- Automated Content Generation: Businesses use generative models to automate content creation for marketing materials, social media posts, and product descriptions.
Conclusion:
Generative AI stands at the forefront of innovation, offering unprecedented capabilities in content creation, image synthesis, and natural language processing. As technology continues to advance, its impact on various industries is likely to grow, unlocking new possibilities for creativity, problem-solving, and human-machine interactions. The future holds exciting prospects as researchers and developers push the boundaries of what generative models can achieve.
To learn about newer in-trend technologies follow our Up-To-Date Blog page written by our expert professionals after successfully implementing and providing solutions to the clients using the same to give our readers more practical insights about the use of technology.
Reach us on:
LinkedIn: https://in.linkedin.com/company/amlgolabs
Website: https://www.amlgolabs.com
Email: [email protected]