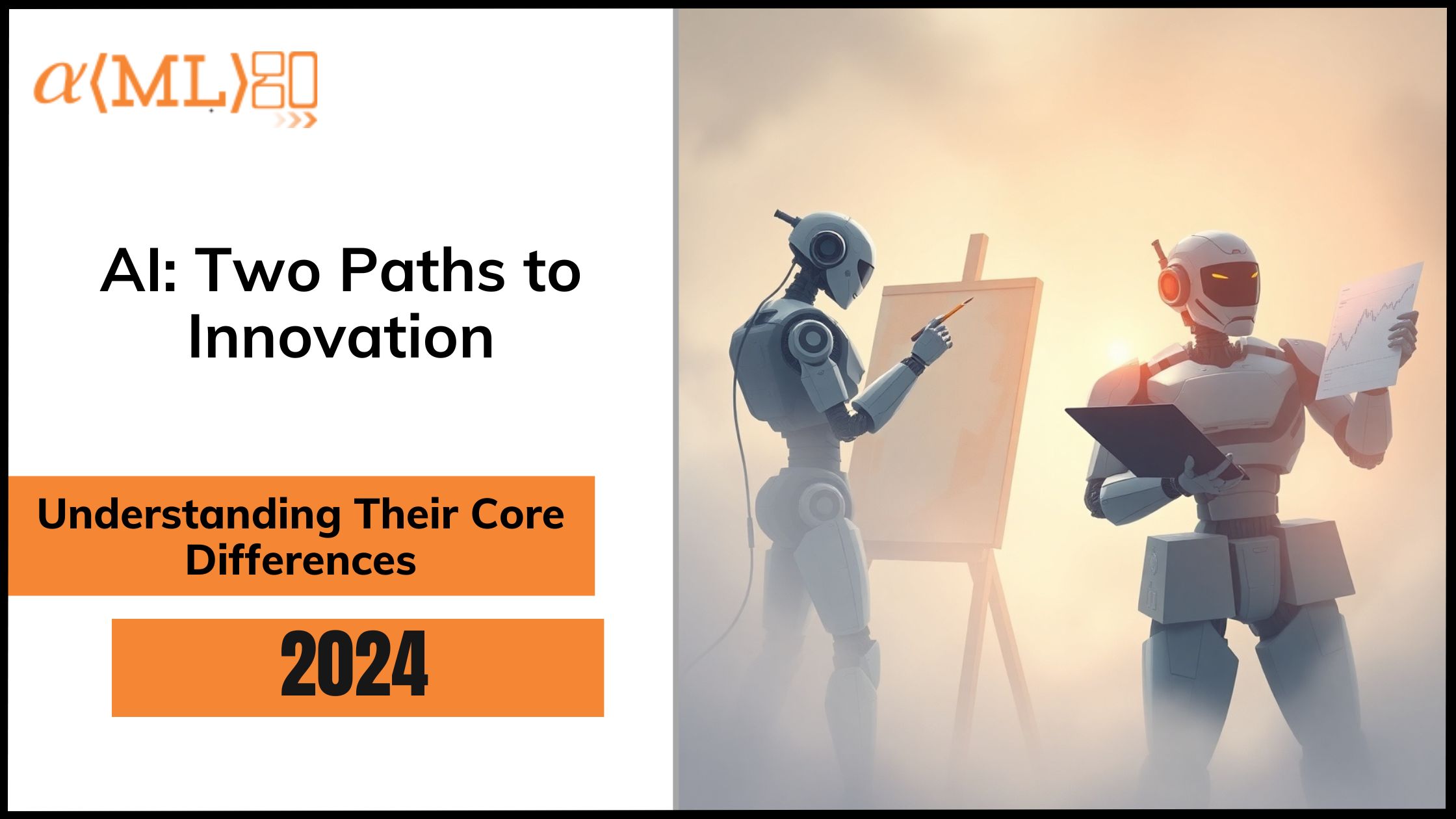
In today’s rapidly evolving landscape of AI for business, companies face a critical choice between AI systems that predict customer behavior and those that create content and responses. This decision highlights an important distinction between generative AI and predictive AI, two technologies that are shaping AI industry trends and driving innovation across sectors.
While these AI technologies belong to the same family, they serve different purposes. Predictive AI analyzes past data to forecast future outcomes, while generative AI creates fresh content ranging from text and images to code and product designs. As AI business applications continue to expand, understanding the nuances between these technologies becomes crucial for effective implementation and measuring ROI.
This comprehensive guide delves into the main differences between these AI technologies and their business uses. You’ll discover implementation strategies, economical solutions specific to various industries, and insights into AI future predictions. This information will help you select the right solution that aligns with your organization’s needs and long-term vision.
Understanding the Business Value Proposition
Before making investment decisions, business leaders need to grasp how predictive and generative AI create value. Let’s examine how these technologies deliver business benefits through different approaches and address varying needs.
Cost-benefit analysis for each AI type
Predictive AI often delivers faster financial returns in day-to-day operations. For instance, UPS saves approximately $35 million annually by using predictive AI to optimize deliveries. Similarly, banks of all sizes can save up to $16 million each year through predictive fraud detection.
These market projections illustrate the value difference:
- The predictive AI market is expected to reach $64 billion by 2025
- Generative AI spending currently represents less than 7% of predictive AI investments
When conducting an AI cost-benefit analysis, it’s essential to consider both immediate returns and long-term potential. While predictive AI may show quicker results, generative AI’s impact on innovation and content creation can lead to significant long-term value.
Resource requirements comparison
Each AI type has unique resource needs that should be carefully considered:
Resource Type | Predictive AI | Generative AI |
---|---|---|
Data Quality | Requires high-quality, structured data | Works with large volumes of varied data |
Processing Power | Lighter computational needs | Intensive computational requirements |
Model Complexity | Dozens to hundreds of parameters | Much larger models |
Implementation Time | Faster deployment for specific tasks | Longer setup and training period |
Understanding these resource requirements is crucial for effective AI implementation and optimizing AI ROI.
Expected ROI metrics
ROI timelines vary between these AI types. Predictive AI projects focusing on operational improvements show returns over time with substantial results. Marketing campaigns utilizing predictive analytics can boost profit up to five times through better customer targeting.
Generative AI requires more upfront investment but adds value across many business functions. Recent studies indicate that generative AI could add between $2.6 trillion to $4.4 trillion annually in various use cases, affecting:
- Customer operations
- Marketing and sales
- Software engineering
- R&D
When measuring AI ROI, it’s important to consider both tangible and intangible benefits. While predictive AI often shows clearer financial returns, generative AI can drive innovation and improve customer experiences, contributing to long-term growth and brand value.
The best returns often come from using predictive AI for specific operational improvements that require accurate forecasting and decision support. Manufacturing companies benefit greatly from this approach, as predictive maintenance reduces downtime and maintenance costs.
Generative AI works best in areas where content creation and innovation drive success. Industries such as banking, high-tech, and life sciences show strong potential. Banks alone could see additional yearly value of $200-340 billion after implementing generative AI.
Decision-Making vs Content Creation
Understanding the core differences between predictive AI and generative AI is crucial for making the right technology decisions for your business. Let’s explore their unique capabilities and how they meet different organizational needs.
Predictive AI’s analytical capabilities
Predictive AI excels at analyzing past data to forecast future outcomes. Implementing predictive AI means utilizing advanced statistical methods to identify patterns and make informed decisions. This technology is at the heart of AI-driven decision making, enabling businesses to anticipate trends and optimize operations.
Research shows that predictive AI creates an autonomy-technology tension that can lower performance expectancy and reduce satisfaction. However, these effects largely depend on how well the AI’s suggestions align with user priorities. To maximize the benefits of predictive AI, it’s crucial to ensure that the system’s outputs are relevant and actionable for your specific business context.
Generative AI’s creative potential
Generative AI can substantially boost your creative processes. Recent research demonstrates that generative AI addresses creativity challenges by:
- Increasing employee and customer creativity
- Blending multiple ideas into stronger solutions
- Supporting divergent thinking through remote concept associations
These capabilities make generative AI particularly valuable for tasks such as content creation, product design, and customer engagement. However, it’s important to note that while AI can increase individual creativity, it tends to make collective output more uniform. Research indicates that AI-assisted content shows more similarities among different creators, which might reduce overall creative diversity.
Choosing the right tool for specific needs
Your specific business objectives should determine whether you opt for predictive or generative AI. Here’s a comparison to help you decide:
Aspect | Predictive AI | Generative AI |
---|---|---|
Primary Focus | Data analysis and forecasting | Content creation and innovation |
Best Use Cases | Financial forecasting, fraud detection, inventory management | Customer service, marketing content, software development |
Data Requirements | High-quality, specific datasets | Large, diverse training data |
The choice between these technologies depends on several factors:
- If innovation is your main goal, generative AI offers better tools for content creation and ideation
- For decision-making and forecasting needs, predictive AI delivers more reliable analytical insights
Data quality and business objectives greatly influence how well your chosen AI solution works. For instance, predictive AI requires high-quality data for accurate predictions, while generative AI needs large datasets to train models effectively.
It’s worth noting that these technologies can complement each other. Forward-thinking businesses often combine both predictive and generative AI to create a comprehensive approach that merges analytical decision-making with creative content generation.
Small Business Implementation Guide
Recent data reveals that 44% of small and medium-sized businesses don’t know where to start with AI adoption. This guide offers a practical approach to help you get started with both predictive and generative AI solutions, addressing common AI implementation challenges.
Starting with limited resources
Successful AI implementation starts small and targets specific business functions. Consider these questions to guide your AI tool investment:
- Which business process needs the most improvement?
- How quickly can your team learn the new technology?
- What results can you measure?
- What return on investment have you predicted?
Start by identifying routine tasks that automation could handle, allowing your team to focus on high-value work. For example, customer service makes an excellent starting point – Wonolo cut their handle times by 20% with AI-powered responses.
Scalability considerations
Your AI implementation plan should include adaptable solutions that grow with your business. Here’s an effective approach:
- Start with controlled experiments
- Measure concrete results
- Scale up usage once benefits become clear
A solid AI strategy should address current business needs while considering integration challenges and staff training. Remember that AI tools support existing processes rather than replacing them entirely. Look for solutions your business can adapt to instead of forcing your business to change for the technology.
Affordable solutions
These proven strategies will help you maximize your AI investment with limited resources:
Strategy | Implementation Approach |
---|---|
Free Trials | Test functionality during trial periods before committing |
Freemium Models | Begin with simple versions and upgrade later |
Cloud-Based Solutions | Cut infrastructure costs while staying adaptable |
AI-enhanced project management tools like Trello, Asana, or ClickUp create efficient teamwork without major investment. Financial management tools like QuickBooks or Wave automate bookkeeping and provide analytical insights into your business’s financial health.
Note that AI implementation doesn’t require a complete system overhaul. You can start with targeted applications that show clear improvements without disrupting current operations. For instance, PaySauce boosted their email open rates from 37% to 52% by using predictive AI to segment customer lists.
Risk Assessment and Management
The integration of AI solutions into business operations demands a clear understanding of risk management for both predictive and generative AI systems. A staggering 96% of leaders believe adopting generative AI increases security breach likelihood, highlighting the importance of addressing these concerns.
Data security considerations
Protection of sensitive information is the top priority for both AI types. Generative AI presents unique challenges because these systems might store input information indefinitely and use it to train other models. Here are the vital security aspects to consider:
Risk Type | Impact | Mitigation Strategy |
---|---|---|
Data Leakage | Exposure of sensitive information | Implement strict access controls |
Privacy Breaches | Unauthorized data access | Regular security audits |
Training Data Security | Model manipulation | Data encryption protocols |
Ethical considerations
Both predictive and generative AI implementations require careful ethical considerations. AI systems can unintentionally create or magnify existing biases, leading to discriminatory outcomes. Studies show that companies without proper governance face substantial legal and financial risks.
Areas that need your focus include:
- Protection against algorithmic discrimination
- Transparency in AI decision-making
- Fair use of training data
- Prevention of unauthorized data access
Compliance requirements
AI implementation must meet evolving regulatory standards. The EU AI Act sets these penalties for non-compliance:
- €35 million or 7% of global annual turnover for prohibited AI system usage
- €15 million or 3% of annual turnover for operational compliance failures
- €7.5 million or 1% of annual turnover for providing inaccurate information to authorities
An effective AI governance strategy helps ensure compliance. Regular system audits confirm alignment with your company’s goals. The risk management framework should address:
- Data privacy regulations and restrictions
- Industry-specific compliance requirements
- Regular system audits and monitoring
- Incident response planning
Staff members who input sensitive data into public generative AI models create major security risks. Clear policies and procedures for AI usage can reduce these risks. Synthetic data helps preserve privacy while maximizing the benefits of machine learning and data analysis.
A thorough risk assessment requires ongoing monitoring of AI-generated content for privacy issues. This becomes vital as 80% of executives plan to invest more in responsible AI approaches to build trust in their models.
Industry-Specific Adoption Strategies
Different industries are discovering innovative ways to leverage both predictive and generative AI technologies. Here’s how three major sectors are using these technologies to address their unique challenges and drive growth.
Healthcare applications
Healthcare organizations can utilize both AI types in various ways. Generative AI shows promising results in drug development, with approximately 70 AI-assisted drugs now in clinical trials. These advances help:
- Speed up drug development timelines
- Cut research costs
- Make clinical trials more efficient
Generative AI tools can write clinical notes and gather information from electronic health records, reducing administrative work and helping prevent staff burnout. AI can also enhance medical imaging by identifying noise in MRI scans and creating cleaner images that lead to more accurate diagnoses.
Financial services implementation
The financial sector has transformed how it uses predictive and generative AI to improve operations. Financial institutions can benefit from these technologies in several ways:
AI Type | Application | Impact |
---|---|---|
Predictive AI | Fraud Detection | Reduces false positives in AML monitoring |
Generative AI | Customer Service | Provides 24/7 customized banking assistance |
Both Types | Risk Management | Improves credit decisions and reduces losses |
Banks see potential annual value increases of $200-340 billion from using generative AI alone, making AI investment a strategic priority for the industry.
Retail and e-commerce solutions
Retail businesses can employ both AI types to create seamless shopping experiences. Major retailers now use AI to:
- Make warehouse logistics more efficient
- Adjust prices in real-time
- Create customized shopping experiences
AI in retail goes beyond simple automation. E-commerce operations can improve through:
- Predictive inventory management that prevents stockouts
- Customer behavior analysis for better merchandising
- Automated checkout systems that speed up service
AI-powered recommendation engines significantly boost sales, with some retailers reporting up to 35% higher conversion rates. Retail businesses can use generative AI to create product descriptions, customer service responses, and marketing content, while predictive AI helps with demand forecasting and inventory optimization.
These industry-specific examples can guide you in choosing the right mix of predictive and generative AI to fit your organization’s needs. Remember that successful AI projects often combine both types rather than relying on just one.
Performance Measurement and Optimization
Effective AI measurement and optimization are crucial for maximizing the value of your AI investments. Different performance measurement approaches are needed for predictive and generative implementations. Organizations that use AI-informed KPIs are 5x more likely to see improved alignment between functions and 3x more likely to be agile and responsive than others.
Key performance indicators
Your AI performance measurement strategy should cover both direct and indirect metrics. Here are effective KPIs for generative and predictive AI applications:
Metric Type | Predictive AI | Generative AI |
---|---|---|
Accuracy | Error rate and precision | Quality index and relevance score |
Speed | System latency | Response generation time |
Efficiency | Resource utilization | Throughput capacity |
User Impact | Adoption rate | User satisfaction score |
Companies that utilize AI-enriched KPIs are 4.3 times more likely to see improved alignment between functions. Focus on metrics that connect to business outcomes while maintaining technical excellence.
Quality assessment methods
A robust quality assessment framework ensures consistent value from AI systems. The QUADAS tool helps assess methodology and has new updates for AI-specific needs. Quality assessment should track:
- Data relevance and reusability metrics
- System integration capabilities
- Model performance stability
- Output consistency and reliability
Research shows 70% of executives believe better KPIs and performance improvements drive business success. Quality assessment needs both technical metrics and business impact measures to provide a comprehensive view of AI performance.
Continuous improvement strategies
Combining AI with Kaizen methods brings major benefits to operational excellence. Your improvement plan should target:
- Data-Driven Optimization
- Results monitoring ensures model performance
- Regular factor reviews identify outdated elements
- Feedback loops enable continuous learning
- Process Enhancement
- Machine Learning analyzes complex patterns
- Natural Language Processing interprets customer feedback
- Predictive analytics forecast system behavior
- Performance Monitoring
- Adoption rates and user engagement tracking
- Session length and query effectiveness measurement
- User satisfaction assessment through surveys and NPS scores
Effective communication and training help address resistance to change. Companies that monitor continuously see better model performance and business results.
Clear objectives and KPI definitions should precede deployment. Track both model quality and system performance to ensure consistent value from AI solutions. Companies using AI to share KPIs are five times more likely to improve alignment between functions.
Note that AI success requires both quantitative metrics and qualitative assessment. Your evaluation should include:
- Content relevance scores for text-based models
- Innovation metrics for creative outputs
- User engagement rates for interactive systems
- System reliability and uptime statistics
These measurement and optimization strategies help maximize value from predictive and generative AI investments. Companies with strong performance monitoring report higher success rates in AI initiatives.
Amlgo Labs: Advanced AI Solutions for Business Success
Amlgo Labs specializes in providing cutting-edge data solutions and AI technologies to empower businesses in the digital age. We offer a range of services, including predictive analytics and generative AI, to help organizations unlock the full potential of their data. Our client base includes industry leaders, demonstrating our expertise and successful track record in delivering transformative solutions across big data, analytics, and forecasting.
Predictive AI Solutions
Predictive AI leverages historical data analysis to forecast future trends and outcomes. This enables businesses to make informed decisions in areas such as:
- Demand Forecasting: Accurately predict future demand for products or services, optimizing inventory and resource allocation.
- Customer Behavior Analysis: Understand customer preferences, predict churn risk, and personalize marketing campaigns for improved customer engagement and retention.
- Risk Management: Identify and mitigate potential risks, such as fraud, credit defaults, and operational disruptions.
Generative AI Solutions
Generative AI focuses on creating new content and experiences. Key applications include:
- AI-Generated Content: Create high-quality content, such as articles, code, and marketing materials, efficiently and cost-effectively.
- Personalized Customer Experiences: Deliver tailored experiences to individual customers, enhancing satisfaction and loyalty.
- Innovation and Creativity: Explore new avenues for innovation and product development by leveraging the creative potential of AI.
Our Approach
- Tailored Solutions: We understand that every business is unique. Our AI solutions are customized to meet your specific needs and objectives, whether it’s predictive analytics, generative AI, or a combination of both.
- Seamless Integration: We prioritize seamless integration of AI solutions into your existing systems, minimizing disruption to your operations and maximizing efficiency.
- Comprehensive Training and Support: We provide comprehensive training to equip your team with the necessary skills to effectively utilize AI technologies. Ongoing support ensures the long-term success and optimal performance of your AI solutions.
- Scalable and Future-Ready: Our AI solutions are designed to scale and adapt as your business evolves. We ensure that your systems remain robust and performant while accommodating future growth and new requirements.
Why you should Partnering with Amlgo Labs
By partnering with Amlgo Labs, you gain more than just a service provider. We become your strategic ally in your AI journey, committed to your success. With our expertise in both predictive and generative AI, we empower you to:
- Make data-driven decisions with greater confidence.
- Unleash creativity and explore new avenues for innovation.
- Redefine what’s possible for your business in the digital age.
Contact Amlgo Labs today to learn more about how we can help you leverage the power of AI to achieve your business goals.
Conclusion
In 2024, businesses can leverage both predictive and generative AI to drive growth. Predictive AI excels at analyzing data to forecast outcomes, informing operational decisions and risk management. Generative AI, on the other hand, fuels innovation by creating new content and experiences, enhancing customer engagement and product development.
The choice between these technologies depends on individual business goals, resources, and industry-specific needs. Many successful organizations combine both approaches for a comprehensive AI strategy.
To maximize AI ROI, focus on clear performance metrics, continuous optimization, and high-quality data. Monitor both technical and business outcomes to ensure consistent value. Small businesses can start with specific applications, while larger enterprises can integrate AI across departments.
Remember, AI enhances existing processes, not replaces them. Successful AI implementation requires careful planning, scalable solutions, and a focus on data security and ethical considerations.
By leveraging industry-specific AI applications and implementing robust performance metrics, businesses can harness the power of both predictive and generative AI to drive innovation, efficiency, and success in the digital age.
FAQs
Q1. What are the key differences between generative AI and predictive AI?
Generative AI creates new content like text, images, or audio, while predictive AI analyzes historical data to forecast future outcomes. Generative AI excels in creative tasks and content creation, whereas predictive AI is better suited for data analysis, decision-making, and forecasting. Amlgo Labs specializes in deploying both generative and predictive AI solutions tailored to your business needs, ensuring you leverage the right technology for maximum impact.
Q2. How do businesses benefit from implementing generative AI versus predictive AI?
Businesses can benefit from generative AI in areas like content creation, customer service, and product innovation. Predictive AI, on the other hand, offers advantages in operational efficiency, risk management, and data-driven decision-making. Many successful organizations implement both types to maximize their digital transformation efforts. Amlgo Labs provides end-to-end AI consulting, helping businesses identify where generative and predictive AI can deliver the most value and implementing customized solutions to achieve measurable outcomes.
Q3. What are the resource requirements for implementing generative AI compared to predictive AI?
Generative AI typically requires larger datasets and more intensive computational power, while predictive AI can work with more structured, specific datasets and has lighter computational needs. Generative AI often involves more complex models with longer setup and training periods compared to predictive AI. Amlgo Labs offers scalable AI solutions, including cloud-based platforms and pre-trained models, enabling businesses of all sizes to implement AI technologies efficiently without overextending their resources.
Q4. How can small businesses start implementing AI with limited resources?
Small businesses can begin by identifying specific processes that need improvement and starting with controlled experiments. They can leverage free trials, freemium models, or cloud-based solutions to test AI functionalities without significant investment. Starting with targeted applications in areas like customer service or project management can deliver noticeable improvements without disrupting current operations. Amlgo Labs provides flexible, cost-effective AI solutions designed specifically for small businesses, helping them identify high-impact use cases and implement AI in manageable phases.
Q5. What are the key performance indicators (KPIs) for measuring AI success in business?
Key performance indicators for AI success include accuracy metrics like error rates and quality indices, efficiency measures such as resource utilization and throughput capacity, and user impact metrics like adoption rates and satisfaction scores. It’s important to focus on metrics that directly tie to business outcomes while maintaining technical excellence. Regular monitoring of both technical metrics and business impact is crucial for ensuring AI solutions deliver consistent value. Amlgo Labs supports businesses in defining relevant KPIs and provides ongoing performance monitoring to ensure AI implementations achieve desired results.