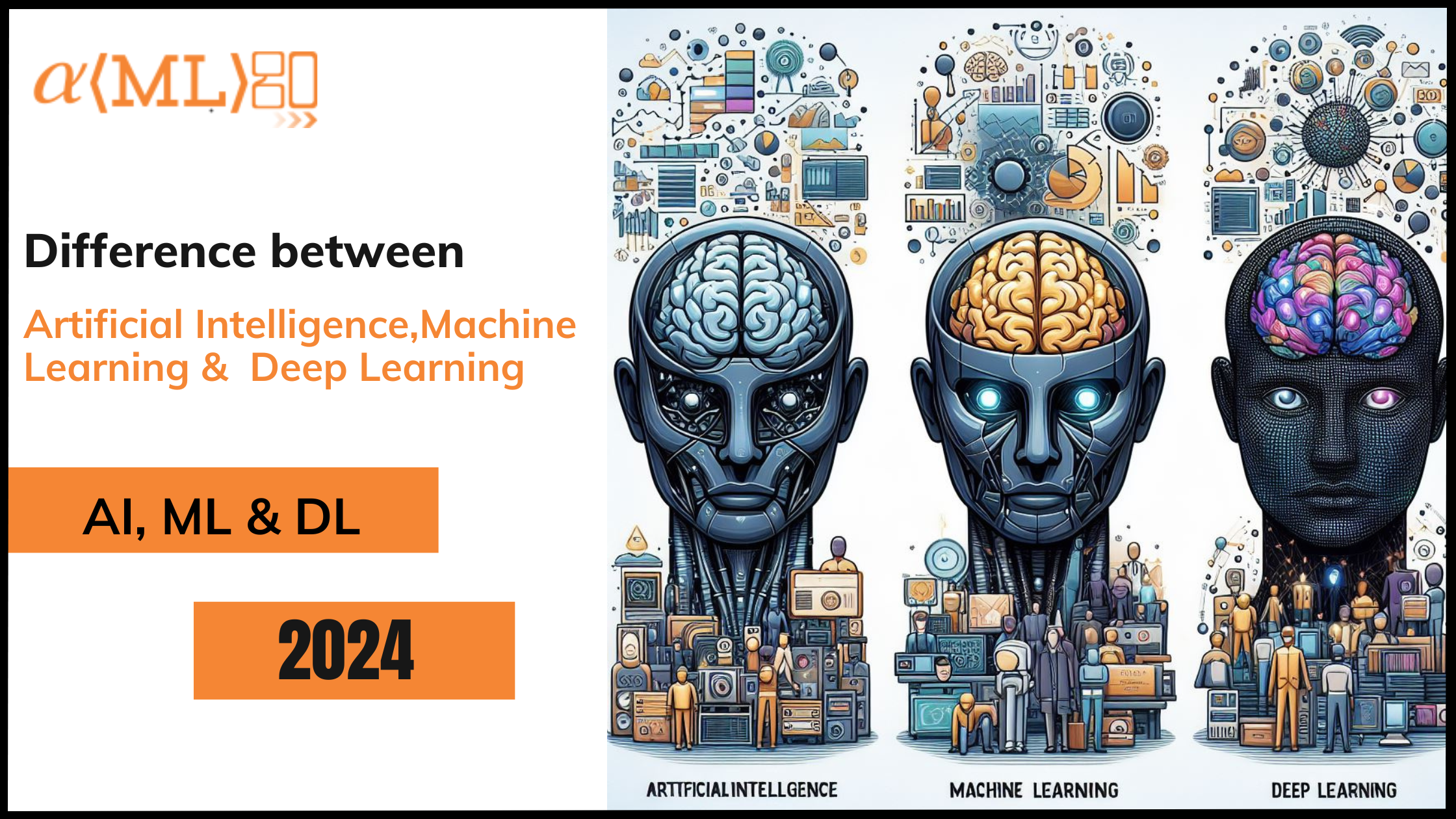
Introduction:
In the fast-paced world of technology, terms like Artificial Intelligence (AI), Machine Learning (ML), and Deep Learning (DL) are frequently thrown around. While they might seem interchangeable, each term represents a distinct layer of the technological landscape. In this blog, we will unravel the complexities and highlight the key differences between AI, ML, and DL, providing you with a comprehensive understanding of these cutting-edge concepts.
Unpacking Artificial Intelligence (AI):
Artificial Intelligence, often abbreviated as AI, is the overarching concept that aims to create machines or systems capable of intelligent behavior. It involves the development of algorithms that enable machines to simulate human-like cognition, decision-making, and problem-solving. AI strives to imbue machines with the ability to learn from experiences, adapt to new situations, and perform tasks that typically require human intelligence.
Demystifying Machine Learning (ML):
Machine Learning is a subset of Artificial Intelligence that focuses on building systems that can learn from data without being explicitly programmed. In other words, ML algorithms enable machines to analyze vast datasets, identify patterns, and make predictions or decisions based on the acquired knowledge. ML is often categorized into supervised learning, unsupervised learning, and reinforcement learning, each catering to specific applications.
Delving into Deep Learning (DL):
Deep Learning is a more specialized subset of Machine Learning that employs neural networks with multiple layers (hence the term “deep”) to analyze and process data. Inspired by the human brain’s structure, deep learning algorithms can automatically learn hierarchical representations of data, extracting intricate features that might be challenging for traditional machine learning models. Deep Learning has proven particularly effective in tasks such as image and speech recognition.
Key Differences:
1 Scope and Purpose:
- AI encompasses the broad goal of creating intelligent machines.
- ML is a specific approach within AI that focuses on data-driven learning.
- DL is an even more specialized area within ML that employs deep neural networks.
2 Learning Mechanism:
- AI can be rule-based or involve learning from experience.
- ML relies on learning from data and improving performance over time.
- DL employs deep neural networks to automatically learn representations from data.
3 Data Dependency:
- AI systems can be rule-based and may not always require extensive datasets.
- ML heavily relies on data for training and improving performance.
- DL, being a subset of ML, also requires significant amounts of labelled data for training.
4 Application Range:
- AI has a broad application range, from robotics to natural language processing.
- ML finds applications in prediction, classification, and clustering.
- DL excels in complex tasks like image and speech recognition.
Conclusion:
In conclusion, while AI, ML, and DL are interconnected, they represent distinct layers of technological innovation. Understanding these differences is crucial for anyone navigating the evolving landscape of artificial intelligence. As we continue to witness advancements in these fields, staying informed will empower individuals and businesses to harness the full potential of these transformative technologies.
Next, we will cover the topic of Generative AI: What is it, Tools, Models, Applications, and Uses Cases.
To learn about newer in-trend technologies follow our Up-To-Date Blog page written by our expert professionals after successfully implementing and providing solutions to the clients using the same to give our readers more practical insights about the use of technology.
Reach us on:
LinkedIn: https://in.linkedin.com/company/amlgolabs
Website: https://www.amlgolabs.com
Email: [email protected]